Imagine a world in which a single tweet could trigger a financial crisis or a deepfake video could cause an international incident. Sounds like science fiction? Think again. These scenarios are becoming increasingly plausible as artificial intelligence (AI) technologies advance. Thanks to advanced AI, it is now disturbingly easy to create convincing fake videos or audio recordings of public figures (Example1 or Example2). While this technology has benign applications in entertainment and education, its potential for misuse in disinformation campaigns is alarming.
The good news? AI is also our best hope for detecting these fakes. Researchers are developing increasingly sophisticated algorithms to recognise the tell-tale signs of synthetic media. But as deepfake technology improves, this cat-and-mouse game is far from over.
The Rise of Synthetic Media: Opportunities and Challenges in the AI Era
In recent years, the world of digital content has been revolutionised by the rise of synthetic media. This cutting-edge technology, based on advanced AI and machine learning (ML) techniques, has opened up new possibilities for data generation, creative expression and entertainment. However, it has also raised significant concerns about potential misuse and the spread of misinformation.
What is Synthetic Media?
Synthetic media refers to artificially generated data, particularly images, videos and audio. This technology has emerged as a solution to the challenges of privacy, efficiency and bias in ML. By creating diverse and representative data sets, synthetic data helps improve AI models’ robustness and fairness.
The Advantages of Synthetic Data
Data augmentation: Synthetic data can complement existing real-world datasets and address issues like scarcity or imbalance. For example, in the development of self-driving car technologies, synthetic data can simulate rare weather conditions that may be underrepresented in real-world data. This augmentation helps to create more comprehensive and diverse training sets for AI models, leading to improved performance and generalisation.
Reduction of bias: Synthetic data can be generated to address and reduce biases in real-world datasets. By carefully controlling the properties of synthetic data, researchers and developers can create more balanced and representative datasets, leading to higher model accuracy, improved model robustness and fairness.
Efficiency and speed: Generating synthetic data is often faster and cheaper than collecting and processing real data, especially for large or sensitive datasets in areas such as healthcare or finance. This efficiency can significantly accelerate research and development cycles, enabling faster innovation and iteration in AI-driven technologies.
Testing and Development: Synthetic data provides a safe environment for testing ML models before deployment, helping identify and fix potential problems without risking sensitive data. This controlled test environment is particularly valuable in high-stakes applications such as medical diagnostics or financial modelling, where errors can have serious consequences.
Data protection: By mimicking real data while protecting individual privacy, synthetic data allows AI models to be developed and tested without jeopardising data confidentiality. This is particularly important in industries that handle sensitive personal data, such as healthcare and finance, where strict data protection regulations apply.
Sample images from three different datasets: a) GTA-V dataset [1] b) Foggy Cityscapes dataset [2]
c) Virtual KITTI dataset [3]
How is Synthetic Data generated?
Several deep-learning techniques are at the forefront of generating synthetic media:
Generative models are a class of ML algorithms trained to learn the underlying probability distribution of the training data. Once trained, they can use this knowledge to generate entirely new data points similar to the original data. Some common generative models for synthetic data are:
- Generative Adversarial Networks (GANs): These consist of two neural networks – a generator and a discriminator – that work together to produce increasingly realistic synthetic data. The generator produces fake patterns, while the discriminator attempts to distinguish between real and fake. This adversarial process leads to a continuous improvement in the quality of the synthetic results [4]. Examples of images of people generated by a type of GAN network can be found here.
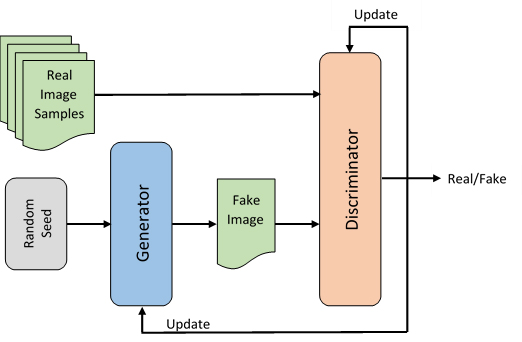
- Variational autoencoders (VAEs): These deep neural network models compress data into a latent space and then reconstruct or generate new data points based on learnt patterns. VAEs are particularly useful for generating different patterns and exploring the underlying structure of the data [5].
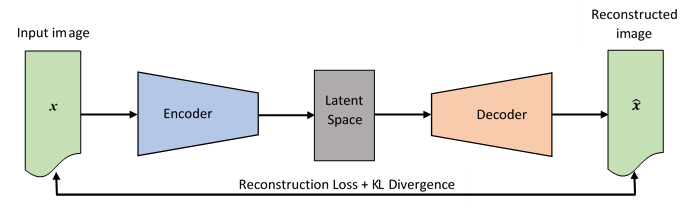
WaveNet models are particularly effective in audio generation. These models predict subsequent audio patterns by analysing sequences of audio information and generating new patterns that closely mimic the target speaker’s voice [6]. This technology has revolutionised text-to-speech systems, enabling more natural and expressive synthetic voices.
ML-based text-to-speech (TTS) systems can generate synthetic speech from text scripts that are often customised to emulate specific voice characteristics [7]. Advanced TTS systems can now generate highly realistic voices with correct intonation and emotion.
Positive applications of synthetic media
Synthetic media has found numerous positive applications in various industries:
Enhanced special effects: The film and gaming industries use synthetic media to create more realistic experiences, from historical re-enactments to fantastical creatures [8]. This technology can be used to create visual effects that would be impossible or prohibitively expensive using conventional means.
Improved accessibility: Synthetic voice generation enables the automatic dubbing of films in different languages, making content more accessible to different audiences [9]. This technology can also help create audiobooks and other voice-based content for people with visual impairments.
Creative expression: Artists and filmmakers are exploring new storytelling techniques and using synthetic media to create unique characters. This opens up new possibilities for creative expression and pushes the boundaries of what is possible in digital art and entertainment [10].
Medical imaging: In healthcare, synthetic media can be used to create realistic medical images for training AI diagnostic systems, which could improve the early detection of disease while protecting patient privacy.
Product design and prototyping: Industries such as automotive and consumer electronics use synthetic media to create realistic 3D models and prototypes to streamline the design process and reduce costs.
The Dark Side: Deepfakes and Misinformation
Despite their benefits, synthetic media, particularly in the form of deep fakes, have raised serious concerns due to their potential for misuse:
Spreading misinformation: Deepfakes can be used to create convincing but fake videos or audio recordings of public figures, which can manipulate public opinion and undermine trust in institutions [11]. This poses a significant threat to democratic processes and social stability.
Damage to reputation: Malicious actors can create fake videos or audio recordings to damage the personal and professional reputation of individuals [12]. The psychological and social impact of such attacks can be devastating and long-lasting.
Financial fraud: Deepfakes of executives could be used to trick employees into authorising fraudulent transactions [13],[14]. As technology becomes more accessible, the risk of sophisticated fraud based on impersonation grows.
Revenge Porn: Non-consensual explicit content can be created by superimposing victims’ faces onto existing material, causing significant psychological damage [15]. This form of abuse raises serious ethical and legal concerns.
Undermining trust: The spread of deepfakes could lead to a general erosion of trust in digital media as people find it increasingly difficult to distinguish between real and fake content.
Fighting Back: AI-Powered Deepfake Detection
To combat the potential misuse of synthetic media, researchers and technology companies are developing various detection methods [16]-[24]:
Anomaly detection: ML models trained on real videos can detect subtle inconsistencies in deepfakes, such as unnatural skin textures or eye movements. These models analyse statistical patterns that occur in real media and highlight deviations from these expected patterns.
Analysing facial features: AI models can detect unnatural movements or inconsistencies in facial features across video frames. This technique is particularly effective in recognising less sophisticated deepfakes, where facial animations can appear unnatural.
Temporal consistency analysis: This technique analyses how light interacts with objects and facial features across multiple frames to detect inconsistencies. It can detect abrupt changes in lighting or shadows that often occur in manipulated videos.
Audio analysis: AI-based tools can identify signatures of synthetic speech generation and recognise inconsistencies in voice patterns or background noise. This is crucial for detecting audio or video deepfakes with manipulated audio.
Deep learning for identifying deep fakes: Interestingly, the same GANs used to generate deep fakes can also be used to recognise them. By training a GAN with real and fake videos, the GAN’s discriminative component learns to recognise subtle flaws in deep fakes. At the same time, the generator refines its ability to create convincing fakes. This adversarial training results in a powerful detection tool that evolves parallel with deep fake techniques.
Blockchain and digital watermarking: New technologies are being explored to authenticate original content as it is created, making it easier to verify the authenticity of media.
Expert analysis: Human experts are still crucial when it comes to recognising subtle signs of manipulation that automated systems might miss. Combining AI-powered tools and human expertise offers the most comprehensive approach to detecting deepfakes.
Ethical and legal considerations
The possibility of creating synthetic media has raised complex questions about copyright and intellectual property, particularly with regard to possible infringements. At the same time, there is an ongoing debate about consent and privacy, with many arguing that explicit permission should be required if a person’s likeness (image/voice) is to be used in synthetic media. In response to these challenges, governments and international bodies are exploring new legal frameworks to address the unique issues posed by synthetic media and deep fakes [25],[26].
A look into the future
As synthetic media continue to evolve, so must our approach to exploiting their benefits while minimising their risks. The continued development of sophisticated detection techniques, combined with greater public awareness and media literacy, will be critical to navigating the complex landscape of synthetic media in the years ahead. By staying informed and vigilant, we can work towards a future where the creative potential of synthetic media is realised without compromising truth and trust in our digital world.
References
- S. R. Richter, V. Vineet, S. Roth, and V. Koltun, “Playing for data: Ground truth from computer games,” in Computer Vision–ECCV 2016: 14th European Conference, Amsterdam, The Netherlands, October 11-14, 2016, Proceedings, Part II 14. Springer, 2016, pp. 102–118.
- C. Sakaridis, D. Dai, and L. Van Gool, “Semantic foggy scene understanding with synthetic data,” International Journal of Computer Vision, vol. 126, pp. 973–992, 2018.
- A. Gaidon, Q. Wang, Y. Cabon, and E. Vig, “Virtual worlds as proxy for multi-object tracking analysis,” in Proceedings of the IEEE conference on computer vision and pattern recognition, 2016, pp. 4340–4349.
- I. J. Goodfellow, J.Pouget-Abadie, M. Mirza, B. Xu, D. Warde-Farley, S. Ozair, A. Courville, and Y. Bengio. “Generative adversarial nets,” in Proceedings of the 27th International Conference on Neural Information Processing Systems, vol. 2, pp. 2672-2680, 2014.
- L. Pinheiro Cinelli, M. Araújo Marins, E. A. Barros da Silva, S. Lima Netto, “Variational Methods for Machine Learning with Applications to Deep Networks”. Springer. pp.111–149, 2021. https://doi.org/10.1007/978-3-030-70679-1_5.
- A. van den Oord, S. Dieleman, H. Zen, K. Simonyan, O. Vinyals, A. Graves, N. Kalchbrenner, A. Senior, K. Kavukcuoglu, “WaveNet: A Generative Model for Raw Audio”, 2016. arXiv:1609.03499.
- V. M. Reddy, T. Vaishnavi, K. P. Kumar, “Speech-to-Text and Text-to-Speech Recognition Using Deep Learning”, 2nd International Conference on Edge Computing and Applications (ICECAA), 657-666, 2023. https://doi.org/10.1109/ICECAA58104.2023.10212222
- AI in the Film Industry: Special Effects and Animation. [Online]. Available: https://thideai.com/ai-in-the-film-industry-special-effects-and-animation/. [Accessed: 21-May-2024].
- Unlock The Future Of Filmmaking With AI for Dubbing. [Online]. Available: https://vitrina.ai/blog/ai-for-dubbing-filmmakers/. [Accessed: 21-June-2024].
- Synthetic Data and AI-created art. [Online]. Available: https://www.linkedai.co/blog/synthetic-data-and-ai-created-art. [Accessed: 21-June-2024].
- How a magician who has never voted found himself at the center of an AI political scandal. [Online]. Available: https://edition.cnn.com/2024/02/23/politics/deepfake-robocall-magician-invs/index.html. [Accessed: 21-May-2024].
- A school principal faced threats after being accused of offensive language on a recording. Now police say it was a deepfake. [Online]. Available: https://edition.cnn.com/2024/04/26/us/pikesville-principal-maryland-deepfake-cec/index.html. [Accessed: 21-June-2024].
- A Voice Deepfake Was Used To Scam A CEO Out Of $243,000. [Online]. Available: https://www.forbes.com/sites/jessedamiani/2019/09/03/a-voice-deepfake-was-used-to-scam-a-ceo-out-of-243000/. [Accessed: 21-June-2024].
- A company lost $25 million after an employee was tricked by deepfakes of his coworkers on a video call. [Online]. Available: https://www.businessinsider.com/deepfake-coworkers-video-call-company-loses-millions-employee-ai-2024-2. [Accessed: 21-June-2024].
- Teen Girls Confront an Epidemic of Deepfake Nudes in Schools. [Online]. Available: https://www.nytimes.com/2024/04/08/technology/deepfake-ai-nudes-westfield-high-school.html. [Accessed: 21-June-2024].
- L. Verdoliva, “Media Forensics and DeepFakes: An Overview”, IEEE Journal of Selected Topics in Signal Processing, vol. 14, no. 5, 2020, pp. 910-93. http://dx.doi.org/10.1109/JSTSP.2020.3002101.
- R. Tolosana et al. “Deepfakes and beyond: A Survey of face manipulation and fake detection”, Information Fusion, vol. 64, 2020, pp. 131-148. https://doi.org/10.1016/j.inffus.2020.06.014.
- S. Agarwal, H. Farid, “Detecting Deep-Fake Videos from Aural and Oral Dynamics”, 2021 IEEE/CVF Conference on Computer Vision and Pattern Recognition Workshops (CVPRW), Nashville, TN, USA, 2021, pp. 981-989. https://doi.org/10.1109/CVPRW53098.2021.00109.
- I. Amerini, A. Anagnostopoulos, L. Maiano, L. Ricciardi Celsi, “Deep Learning for Multimedia Forensics”, Foundations and Trends in Computer Graphics and Vision, vol. 12, no. 4, 2021, pp. 309-457. http://dx.doi.org/10.1561/0600000096
- R. Tolosana et al. “DeepFakes Evolution: Analysis of Facial Regions and Fake Detection Performance”. Pattern Recognition. ICPR International Workshops and Challenges, 2021. https://doi.org/10.1007/978-3-030-68821-9_38
- S. Zobaed et al. “DeepFakes: Detecting Forged and Synthetic Media Content Using Machine Learning”, Artificial Intelligence in Cyber Security: Impact and Implications. Advanced Sciences and Technologies for Security Applications. Springer, 2021. https://doi.org/10.1007/978-3-030-88040-8_7.
- A. Qureshi, D. Megías and M. Kuribayashi, “Detecting Deepfake Videos using Digital Watermarking,” 2021 Asia-Pacific Signal and Information Processing Association Annual Summit and Conference (APSIPA ASC), Tokyo, Japan, 2021, pp. 1786-1793.
- S. Agarwal, L. Hu, E. Ng, T. Darrell, H. Li, A. Rohrbach, “Watch Those Words: Video Falsification Detection Using Word-Conditioned Facial Motion”, 2023 IEEE/CVF Winter Conference on Applications of Computer Vision (WACV), Waikoloa, HI, USA, 2023, pp. 4699-4708. https://doi.org/10.1109/WACV56688.2023.00469.
- Deepfakes and Blockchain. [Online]. Available: https://struckcapital.com/deepfakes-and-blockchain/. [Accessed: 21-June-2024].
- M. Gal, O. Lynskey, “Synthetic Data: Legal Implications of the Data-Generation Revolution, 109 Iowa Law Review, Forthcoming, LSE Legal Studies Working Paper No. 6/2023, 2023. http://dx.doi.org/10.2139/ssrn.4414385
- Understanding Synthetic Data, Privacy Regulations, and Risk: The Definitive Guide to Navigating the GDPR and CCPA. [Online]. Available: https://cdn.gretel.ai/resources/Gretel-Understanding-Synthetic-Data-and-GDPR.pdf [Accessed: 20-August-2024].
Author
Prof. Emmanuel Karlo Nyarko, PhD
Text and/or images are partially generated by artificial intelligence