As the global population surpasses 8 billion, the demand for food continues to rise sharply. According to projections, we will need to produce 70% more food by 2050 [1], placing immense pressure on agricultural systems worldwide. However, the agriculture sector faces an escalating dilemma: a significant shortage of labour [2]. Many agricultural workers are ageing, younger generations are migrating to urban areas for better opportunities, and fewer individuals are entering the agricultural workforce. This combination of increased demand and reduced supply creates a pressing need for innovation, particularly through automation and advanced technologies such as AI-powered agro robots.
The Motivation: Addressing Food Demands Amid Labor Shortages
The growing gap between food demand and agricultural labour supply is becoming increasingly evident. The ageing farming population and the disenchantment of younger generations with the agricultural lifestyle means fewer people are available to tend to fields. In many countries, farmers are retiring without successors, leading to skills gaps that threaten agricultural production.
In response to these accumulating pressures, the agriculture industry must embrace technological advancements to remain viable. What was once considered a labour-intensive industry must transition toward a model that integrates mechanization, robotization and AI to address these labour shortages effectively.
Mechanization vs. Robotization vs. AI-Driven Robotics
Historically, mechanization involved the use of machinery to assist in various agricultural tasks, such as tractors and harvesters. While mechanization increased efficiency and productivity, it still relied heavily on human intervention for operation and decision-making.
Robotization, on the other hand, refers to the development of machines capable of performing specific tasks autonomously. This includes robotic arms in greenhouses or automated seed planters—devices primarily focused on task efficiency.
However, the ultimate transformation lies in AI-driven robotics, which incorporates artificial intelligence to make decisions in real-time. These systems not only execute predefined tasks but can learn and adapt to their environments, making them pivotal for farming in less predictable and dynamic conditions. For example, AI-powered robots can identify pests, assess crop health, and even determine the best time to harvest—all autonomously.
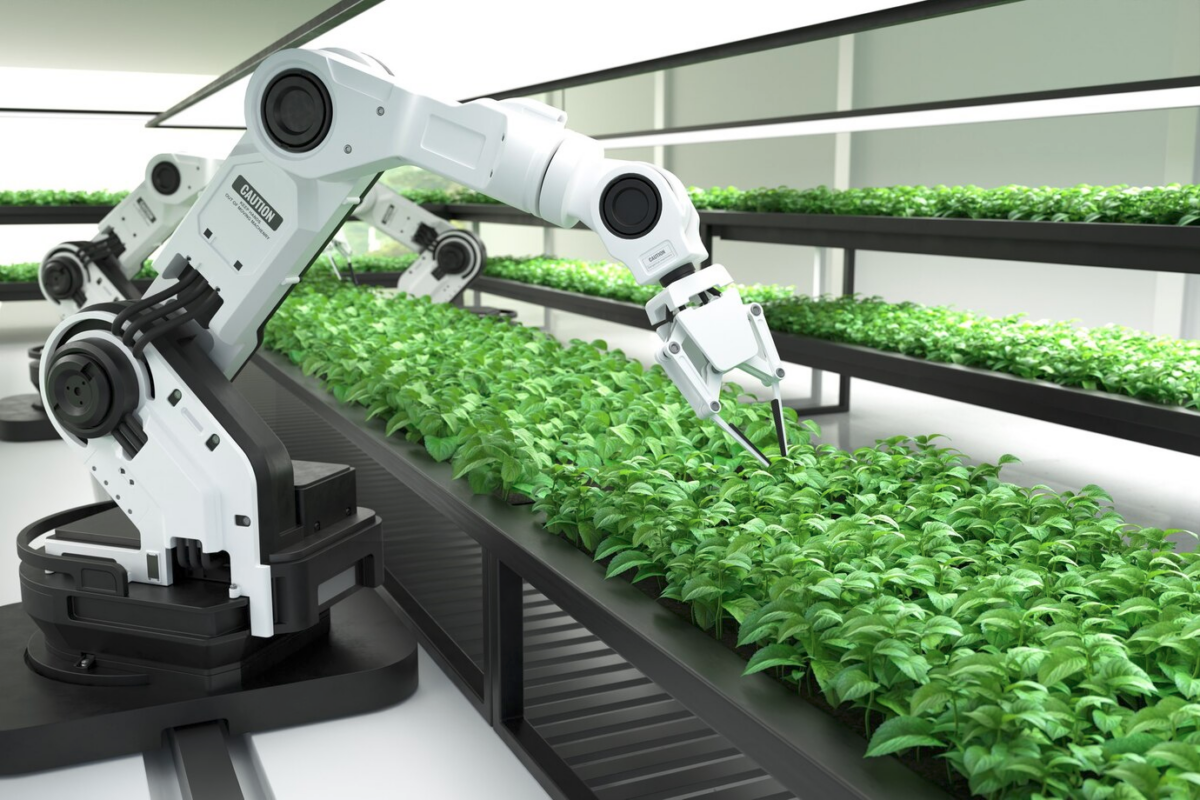
Types of Agricultural Robots
Within the realm of agricultural robotics, several notable types emerge based on their specific purposes [3]:
- Monitoring Robots: These robots use sensors and cameras to gather data about crop health, soil conditions, and other environmental factors. UAVs (drones) are particularly popular for aerial monitoring.
- Harvesting Robots: Designed to identify ripe crops and harvest them, these robots are increasingly becoming automated systems that increase the efficiency of harvesting operations.
- Pruning Robots: These robots assist in maintaining plant health by cutting away dead or diseased branches, helping to promote growth.
- Weeding Robots: Equipped with advanced identification algorithms, these robots can distinguish between crops and weeds, allowing them to effectively eliminate unwanted plants without damaging the crops.
- Pest Control Robots: Utilizing sensors and AI, these robots can identify and target pests, minimizing pesticide use and promoting sustainable farming practices.
- Livestock Robots: Used for herding and monitoring the health of livestock on large ranches.
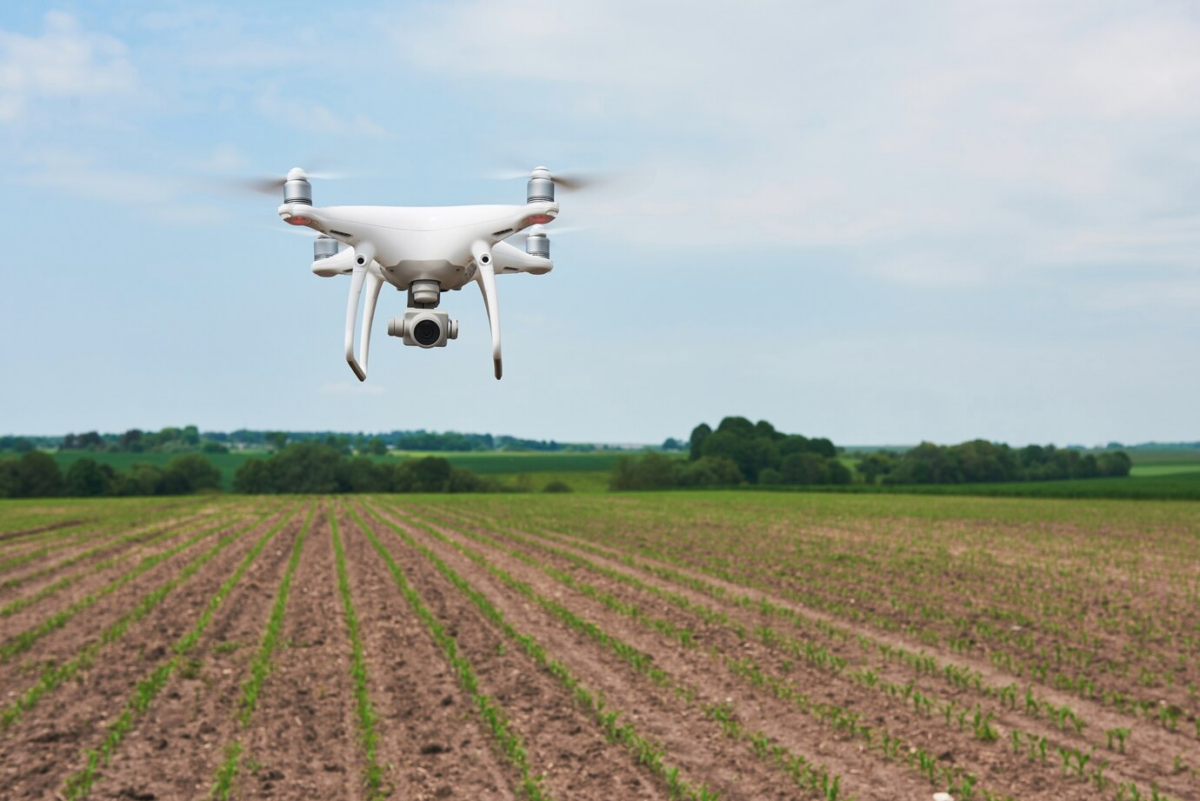
The Growing Agro Robot Market
The global market for agricultural robots is witnessing rapid growth, with a significant increase in investment as companies recognize the potential for both production efficiency and sales. In 2024, the market value is estimated at $16.6 billion, with projections indicating it could reach $51 billion by 2029 [1]. This expansion is driven by various factors, including the increasing demand for food, the necessity to optimize resource utilization, and technological advancements in robotics and AI.
This growth isn’t limited to just robots; it extends to the essential hardware components like batteries, navigation systems, and sensors, as well as the software that powers them. Algorithms based on machine learning and artificial intelligence play a crucial role in the functionality of these systems, particularly in areas such as recognition, localization, navigation, and path planning. Many of these algorithms are built on neural networks, enabling the systems to process large sets of data, learn from their environments, and make intelligent decisions.
The Complexity of Real-World Implementation
Despite the promising advancements showcased in commercials and online videos, the reality of deploying fully autonomous robots in the field is far from straightforward. Various challenges must be addressed before these systems can operate effectively in agricultural environments.
- Territorial Challenges: The terrain on farms can often be unstructured and muddy, complicating the autonomous navigation of robotic platforms. Unlike smooth laboratory floors, real fields present irregularities such as uneven ground, ditches, rocks, and even crop residues that require sophisticated navigation systems to handle obstacles seamlessly. For instance, a robotic weed removal system must adapt its path to avoid large stones, while also maneuvering around plants that are irregularly placed due to natural growth patterns. Robotics companies are increasingly turning to advanced sensors like LiDAR and 3D cameras to help these machines navigate complex terrains. In addition to advanced navigation systems, the hardware components of these robots, such as wheels and tracks, play a crucial role in their ability to traverse difficult landscapes. Wheels need to be exceptionally robust and designed to handle a variety of terrains—from soft, muddy fields to hard, uneven surfaces—without slipping or getting bogged down. Some robots utilize large, wide wheels or tracks to distribute weight and improve stability, helping them maintain grip on slippery surfaces.
- Environmental Impacts: Weather conditions significantly affect the performance and longevity of agricultural robots. Rain, for instance, can create muddy conditions that make the mobility of wheeled or tracked robots extremely difficult. Additionally, inclement weather can lead to the accumulation of water on optical sensors, which might obscure sight and mislead the robot’s navigation systems, ultimately causing operational failures.
Cold weather poses its own set of challenges. Low temperatures can lead to freezing components, potentially causing mechanical failure in motors and joints, while battery efficiency can drastically decrease, reducing operational time. For example, lithium-ion batteries, commonly used in robotic systems, experience diminished performance in cold conditions, which directly impacts the robot’s ability to function effectively during crucial periods such as planting or harvesting. Components such as sensors and electronic circuitry can also be affected, as freezing temperatures can lead to brittle materials that are more prone to breakage or malfunction.
In contrast, hot weather can lead to overheating, particularly in robotic systems that run intensive computational algorithms for navigation and data processing. Extended exposure to high temperatures can result in thermal throttling, where the robot scales back its performance to prevent damage. This issue may hinder the robot’s response time and processing capabilities, which is critical for tasks that require real-time data analysis, such as pest detection or crop health monitoring. Furthermore, heat can affect the effectiveness of various sensors, causing inaccuracies in readings and impairing the robot’s performance. For instance, bright sunlight can cause glare that interferes with the operation of vision sensors, impacting the robot’s ability to identify and track objects accurately.
To combat these environmental challenges, agricultural robots could be designed with temperature-resistant materials and advanced cooling systems. - Computational Power: Running algorithms in real-time necessitates robust computational capabilities. However, it is challenging to equip mobile robots or drones with powerful GPUs due to size, weight, and power supply constraints. For example, a state-of-the-art AI model for computer vision requires considerable processing power, which is difficult to achieve within the limited space of a farm vehicle. Consequently, many robotic systems rely on remote processing, which introduces challenges such as latency and dependence on stable internet connectivity. This limitation is particularly pronounced in rural farming areas with poor connectivity, where uploading data for cloud processing may not be feasible.
- Dynamic Nature of Agriculture: The agricultural landscape is inherently dynamic; plants grow, pests appear, and environmental conditions change daily. For example, what was a clear path in the morning may become obstructed by newly fallen branches or weeds by the afternoon. These variations can confuse recognition algorithms that have not been sufficiently trained on diverse datasets. If a robot is trained primarily on images from sunny weather, it may struggle to identify crops in overcast or rainy conditions.
Collecting standardized datasets suitable for training these algorithms is crucial yet challenging, often requiring substantial time and resources. Data collection might involve lengthy periods of observation across different seasons and weather patterns—tasks that are labor-intensive and costly. Some companies are employing simulation software to create synthetic training data, allowing algorithms to learn from virtual environments before being deployed in the real world. - Maintenance: Agro robots require regular maintenance to ensure optimal performance and longevity. This includes routine checks of their mechanical systems, software updates, battery management, and repairs for wear and tear. As a result, these maintenance needs create new job opportunities in the agricultural sector, such as technician roles focused on robot upkeep, software specialists for programming and troubleshooting, and educators or trainers to guide farm workers in operating and maintaining the technology.
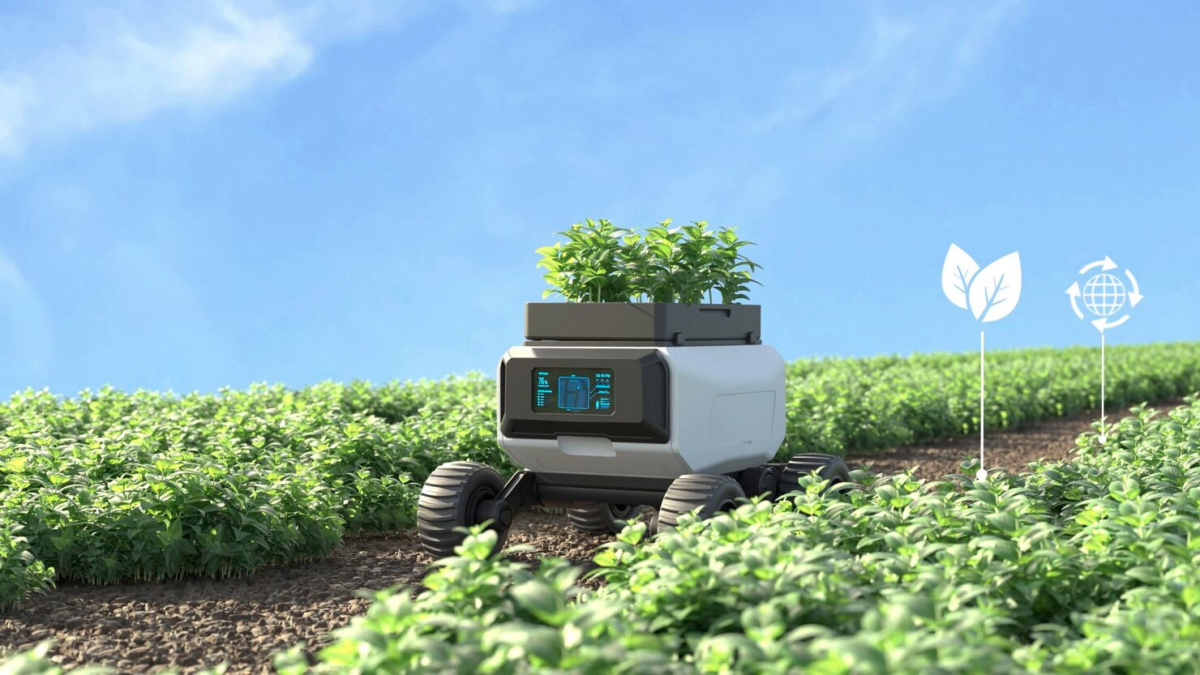
Ethical Considerations in Agro Robotics
The rise of AI-powered agro robots brings forth important ethical considerations that must be addressed as the agricultural landscape evolves. One key area of concern is the interaction between robots and human workers or animals [4]. As these machines become more integrated into farming practices, there is an increased need to evaluate how they operate around people. Unintended interactions—such as a robot miscalculating its movements during a task—can pose risks to farmworkers and bystanders. For instance, a robotic harvester operating in a crowded field could potentially harm a worker, an animal or damage property if its navigation systems fail or if it misinterprets its surroundings.
To mitigate such risks, robotic systems must be equipped with advanced sensors and safety features that allow them to detect and respond to human presence. Innovations such as emergency stop mechanisms, collision detection systems, and fail-safe protocols are essential in ensuring that robots can operate safely in shared environments. Moreover, providing clear communication signals to human workers when robots are in operation can help minimize the likelihood of accidents, fostering a safer coexistence on the farm.
Equity in access to technology is also essential for fostering a sustainable and inclusive agricultural future [5]. Advanced robotic systems can be expensive, and small farms and family-run operations may find it challenging to afford such innovations. This financial barrier could further lead to inequality of farming, where larger corporations with greater financial resources thrive while smaller entities struggle to stay afloat. The reliance on automated solutions may favor large-scale industrial operations that can invest in cutting-edge technologies, potentially marginalizing smallholders.
Another ethical consideration revolves around the environmental impact of robotics. While many agro robots are designed to optimize resource use—such as reducing water and pesticide usage—there is a need to assess the life cycle impact of manufacturing, maintaining, and eventually disposing of these machines. Robotics that prioritize efficiency must also be evaluated for their ecological footprint, including the sourcing of materials and energy consumption. The principles of sustainability must guide the development of agro robotics to ensure they contribute positively to the environment rather than exacerbate existing issues.
Finally, the data privacy and ownership issues surrounding AI technologies can also pose ethical dilemmas [5]. As farming technology increasingly relies on data collection and analysis, questions arise about who owns the data generated by agricultural robots and how it is used. Farmers may become reliant on proprietary systems that lock them into specific vendors, potentially leading to exploitation or unequal power dynamics in the farming industry.
These ethical considerations underscore the necessity for inclusive policymaking and community engagement as the relationship between agriculture and technology evolves. By addressing these issues thoughtfully, the agricultural sector can harness the benefits of automation while safeguarding the interests of workers, small farmers, and the environment.
Innovation and Government Support
Despite the challenges, the potential for innovation in agro robotics remains robust. Many governments and organizations are recognizing the need for investment in agricultural technology [6-8]. Funding for research and development is increasingly available, aimed at fostering advancements that will enhance productivity while addressing the unique challenges of agriculture.
Scientific contributions in both hardware and software design are vital. Researchers are exploring novel ways to create more adaptable sensors, better data algorithms, and efficient energy sources that can overcome the limitations of current systems. The collaborative effort between academia, industry, and government is critical to developing solutions that can effectively meet the agricultural labor gap.
The Future of Agro Robots: Efficiency and Feasibility
The crucial question remains: can these advancements genuinely replace human labor? While fully autonomous systems have the potential to operate continuously, day and night, making them significantly more efficient than traditional farming methods, several factors must be considered.
First, the specific tasks that agro robots may be capable of completing autonomously will likely be limited. While robots can excel at defined functions like planting, weeding, and monitoring, many agricultural tasks require human judgment, intuition, and adaptability that machines currently can’t replicate.
Furthermore, the efficiency of these systems depends on their ability to work seamlessly with existing agricultural practices. Farmers often have unique strategies honed over years of experience, and robotic systems must integrate into these practices without being overly disruptive.
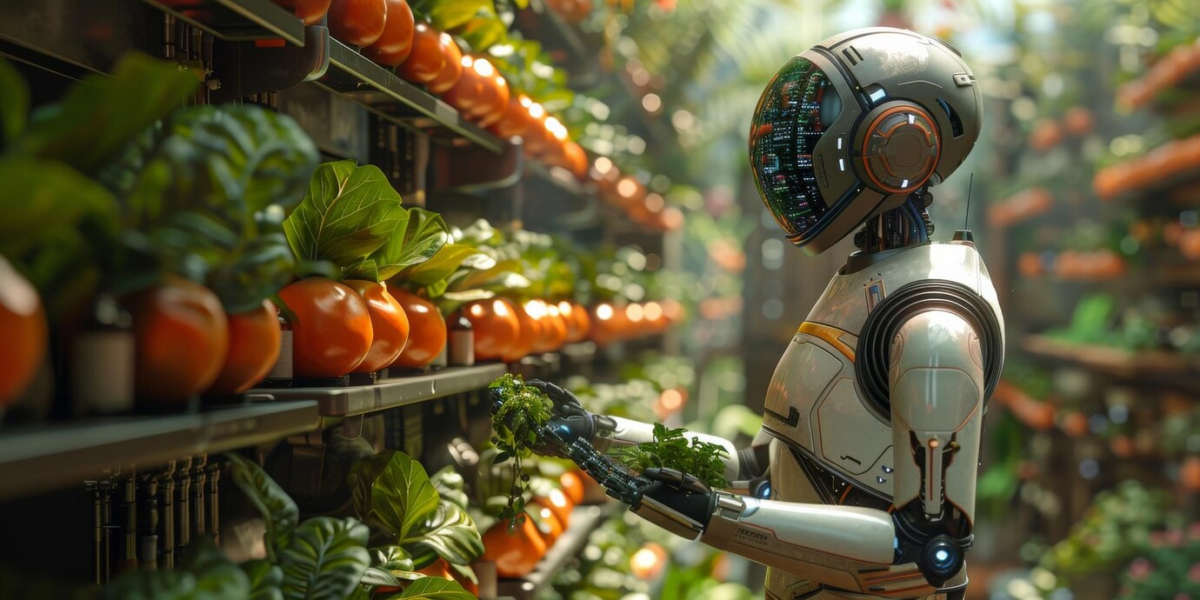
Conclusion: The Potential of Targeted Solutions
As we stand at the crossroads of technological advancement and agricultural necessity, the future of agro robots promises to deliver innovative solutions designed for specific tasks. While these technologies may not completely replace the human touch in farming, they can complement and enhance traditional methods, maximizing agricultural productivity while addressing labor shortages.
Moving forward, it is vital to balance innovation with ethical considerations, ensuring that all stakeholders—including small farmers—have the opportunity to thrive in this new era of agriculture. With continued investment in research and support for technological integration, AI-powered agro robots can help cultivate a more efficient, sustainable, and equitable future for food production.
[1] “How Farm Robots Are Changing The Future of Agriculture.” Accessed: Feb. 18, 2025. [Online]. Available: https://www.agrirs.co.uk/blog/2022/06/how-are-farm-robots-shaping-the-future-of-agriculture?source=google.com
[2] “Agriculture Robots Market Industry Analysis | Types, Advantages, and Forecast,” MarketsandMarkets. Accessed: Feb. 18, 2025. [Online]. Available: https://www.marketsandmarkets.com/Market-Reports/agricultural-robot-market-173601759.html
[3] “Exploring the Future of Agriculture: A Deep Dive into Robots.” Accessed: Feb. 18, 2025. [Online]. Available: https://www.agritecture.com/blog/exploring-the-future-of-agriculture-a-deep-dive-into-robots
[4] University of Reading, UK, K. Ayris, D. C. Rose, and Cranfield University, UK, “Social and ethical considerations for agricultural robotics,” in Burleigh Dodds Series in Agricultural Science, Wageningen University, The Netherlands and E. Van Henten, Eds., Burleigh Dodds Science Publishing, 2024, pp. 513–530. doi: 10.19103/AS.2023.0124.20.
[5] R. Dara, S. M. Hazrati Fard, and J. Kaur, “Recommendations for ethical and responsible use of artificial intelligence in digital agriculture,” Front. Artif. Intell., vol. 5, p. 884192, Jul. 2022, doi: 10.3389/frai.2022.884192.
[6] “Funding available for robotics and automation in farming – Farming.” Accessed: Feb. 20, 2025. [Online]. Available: https://defrafarming.blog.gov.uk/2022/12/13/funding-available-for-robotics-and-automation-in-farming/
[7] P. Hahnloser, “Federal initiative seeks research into agricultural robots,” Government Market News. Accessed: Feb. 20, 2025. [Online]. Available: https://govmarketnews.com/federal-initiative-seeks-research-into-agricultural-robots/
[8] S. Revankar, “Agricultural Robotics Statistics By Revenue, Drones, Milking Robots and Facts,” Coolest Gadgets. Accessed: Feb. 20, 2025. [Online]. Available: https://www.coolest-gadgets.com/agricultural-robotics-statistics/
Author
Assistant prof. Petra Pejić, PhD
Text and/or images are partially generated by artificial intelligence
Images source: freepik.com