What is biometrics?
Biometrics is an interdisciplinary field of science that deals with the measurement and analysis of unique physical and behavioural characteristics of humans for the purpose of identification or verification of identity. Identification involves matching biometric data against a database to determine identity (“Who are you?”), while verification confirms if biometric data matches a particular person’s record (“Are you who you say you are?”). But what we should rely on for dealing with such tasks? You probably know that your fingerprints are unique. However, there are many other physical attributes of your body such as the pattern of the iris of your eyes or the shape of your ears that can be used for biometric tasks. In addition to physical characteristics, many modern biometric systems also rely on behavioural characteristics such as your speech pattern or even your gait. The advantage of biometric authentication lies in its security and ease of use. Instead of juggling passwords and PINs that can easily be forgotten or compromised, biometric authentication offers a natural and more secure solution – something that is part of you becomes your key. Modern biometric systems, despite their advancement, certainly aren’t foolproof and have their limitations when it comes to security. However, artificial intelligence (AI) methods are pushing the boundaries of robustness, security and accuracy of modern biometric systems that are becoming a part of our everyday lives. Let’s take a quick look at some examples.
What are Deep Neural Networks?
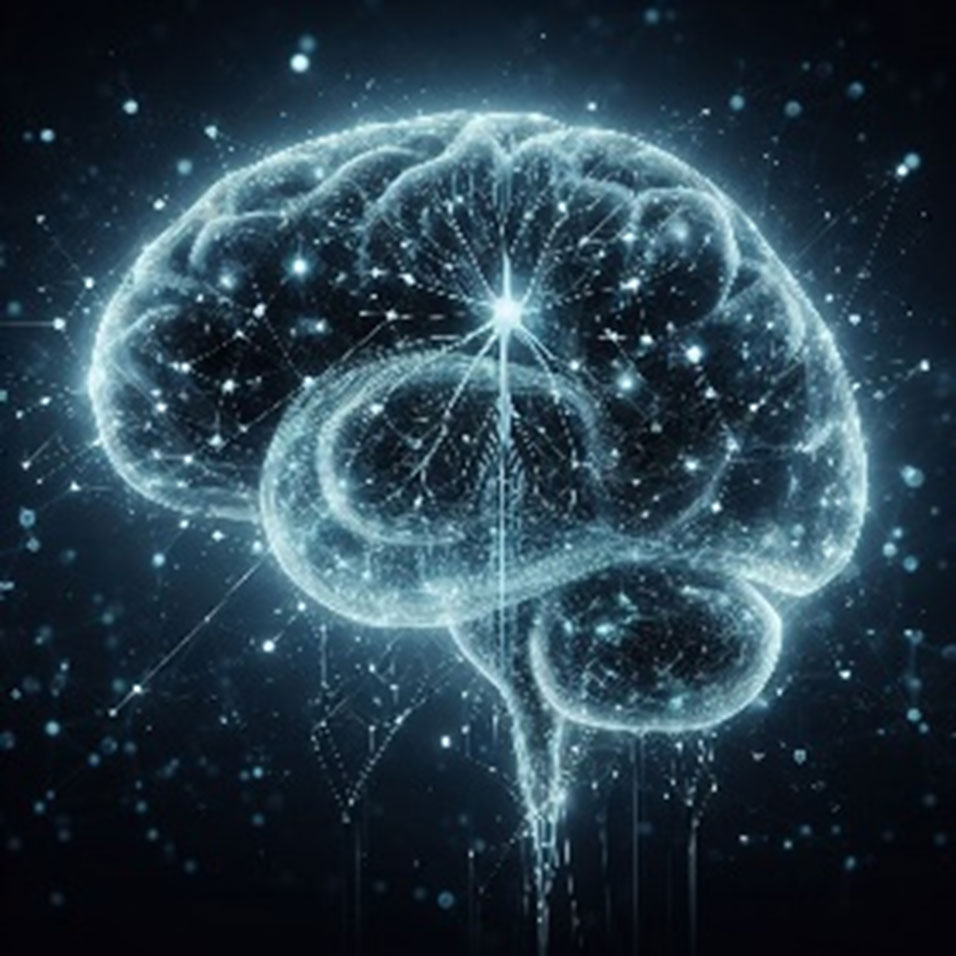
Deep Neural Networks (DNNs), a common form of AI, are revolutionizing biometrics. DNNs are essentially algorithms composed of multiple layers of interconnected artificial neurons. These artificial neurons process information, in a similar way to the biological neurons in our brains. Each layer performs specific computations on the data it receives until the desired output is achieved. We can train such networks for a specific task by using labeled data. With each iteration during this training process, the DNN refines its internal connections, so that it can recognize patterns and make increasingly accurate predictions.
The Power of AI in Biometrics
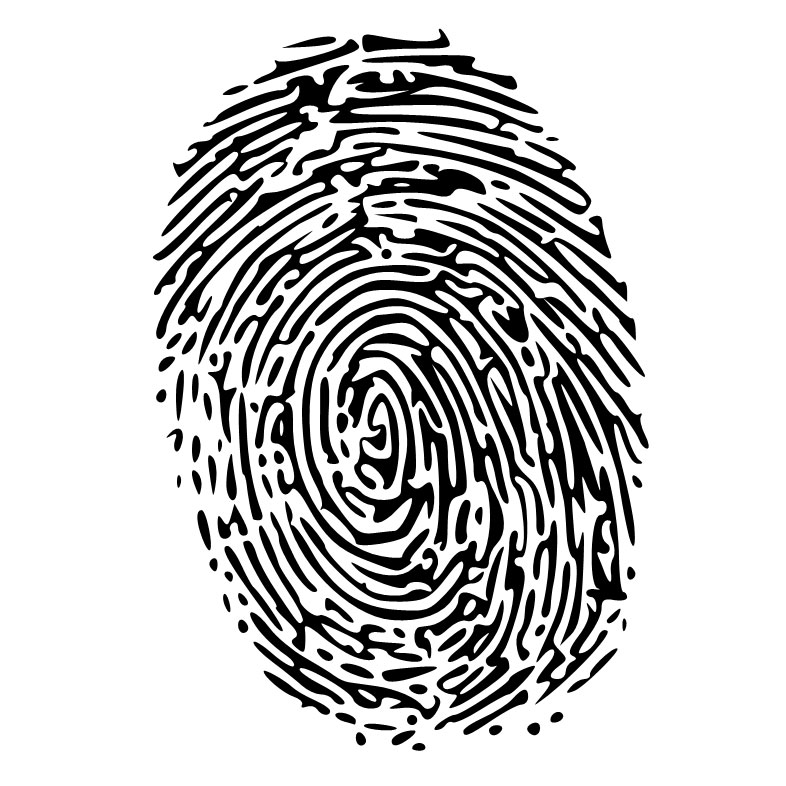
Have you ever wondered why you must provide your fingerprints to the authorities when you get your ID card? Fingerprint recognition is probably the most widely used identification method at the moment due to the reliability of finger patterns over a long period of time. Conventional fingerprint recognition often relies on template matching. We tap our fingers on our phones without a thought, but fingerprint scanners are surprisingly complex under the hood to provide a satisfactory level of security. For example, your smartphone fingerprint sensor (optical, capacitive or ultrasonic) captures an image of your fingerprint when you first set up fingerprint unlock. This image is then converted into a mathematical representation, essentially a unique fingerprint “code,” which is securely stored on your device. During fingerprint recognition, a new scan of your fingerprint is compared to the stored template. A high degree of similarity between the two allows device unlocking. Recently, many scientific papers are incorporating Convolutional Neural Networks (CNNs), specific type of DNNs that excel at image recognition tasks, to enhance fingerprint recognition. They can analyze the intricate details and variations within your fingerprint, leading to improved accuracy compared to template matching alone. Additionally, deep learning can be used to detect finger presentation attack by classifying fingerprints as live/non-live. For example, spoofing attempts can be made using 2D/3D printing or with gummy fingers [1].
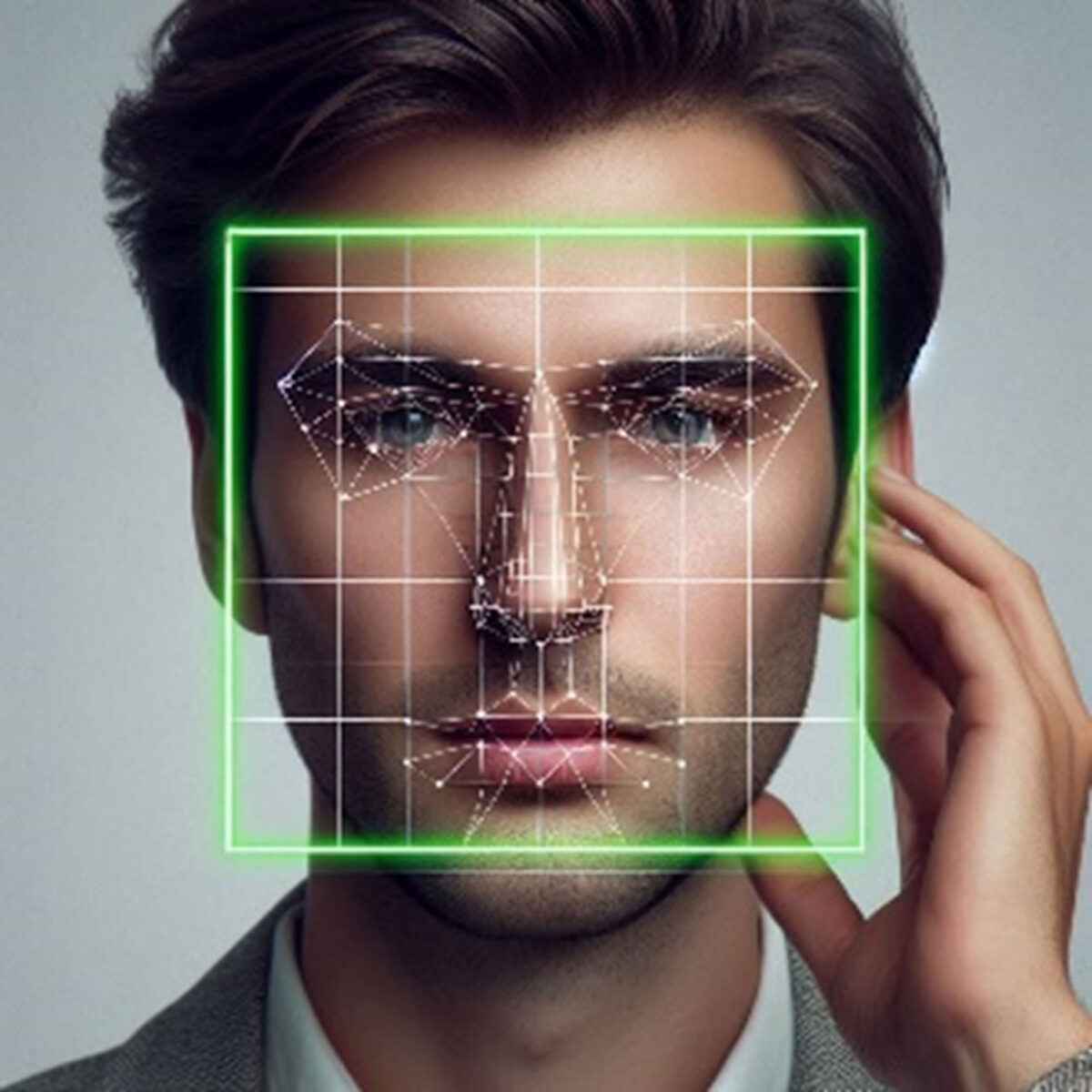
Let’s take a brief look at face detection problem. The problem can be defined as the localization of human faces within a digital image or video frame using rectangular bounding boxes. Although face detection isn’t technically a biometric authentication method, it is used in many biometric systems that rely on facial characteristics. For example, the earliest Apple software solution was based on obsolete Viola-Jones algorithm from traditional computer vision which has poor performance in challenging environments when compared to modern deep learning approaches. Apple started to use DNNs for face detection with iOS10 back in 2016. Specifically, Apple used a fully convolutional deep neural network for face detection. However, such a complex network had unacceptable performance and power usage on iPhone with significant memory requirements. Therefore, engineers created a student network that mimicked the behaviour of a more complex network (so called teacher-student training in machine learning). They were able to achieve state-of-the-art accuracy with such compact network, and with additional memory and GPU optimizations, the detector ran smoothly in real-time on iPhones [2]. While we take face detection on our smartphones for granted today, this was an amazing engineering work back then.
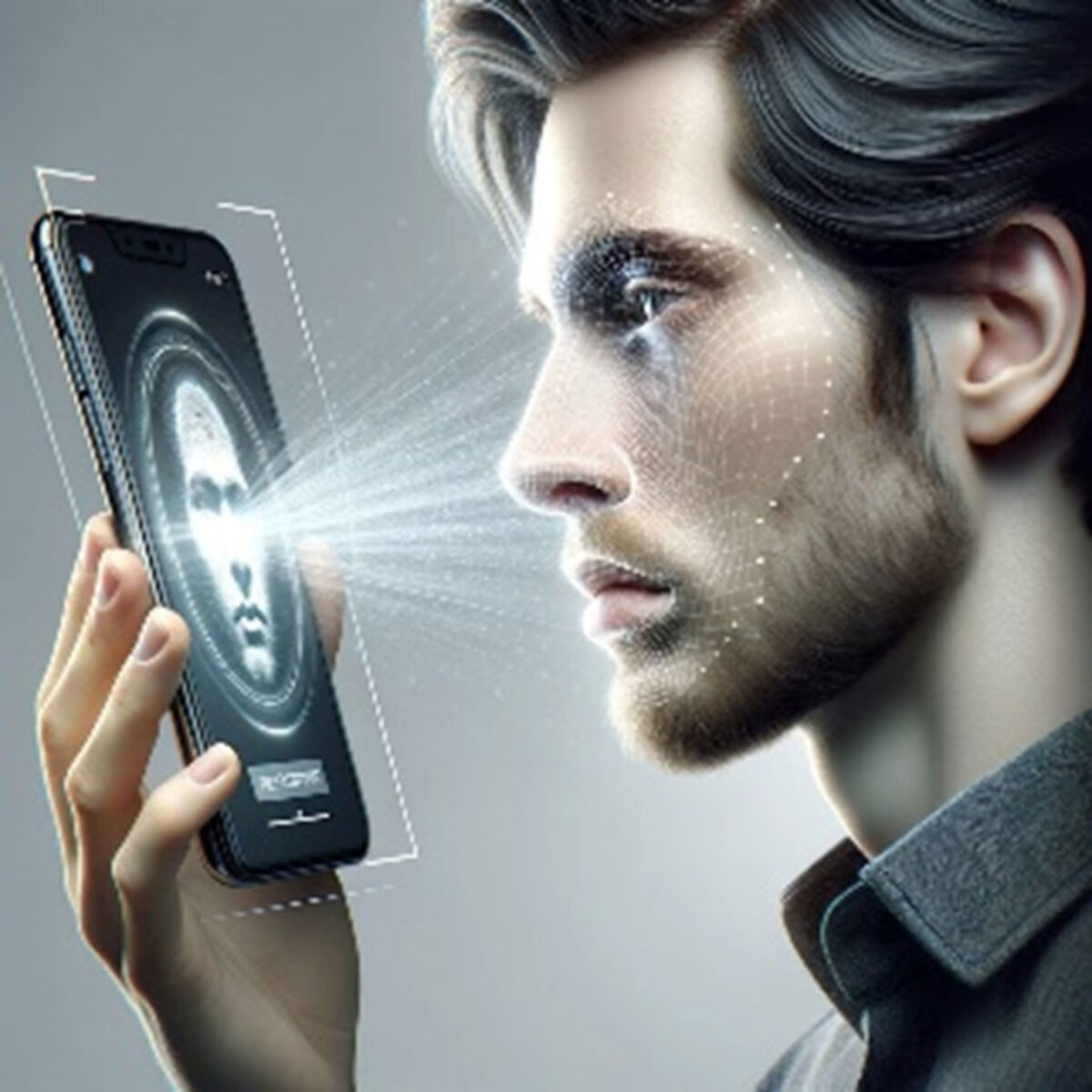
Face recognition is another popular technology in modern biometric applications. Unlike fingerprint scanning, face recognition is contactless. There are no problems with sweaty or dirty hands as with fingerprint recognition. In practice, you only need to look at a camera for identification. Not to mention that many devices/systems already have a built-in camera, making face recognition a cost-effective solution. The pipeline in case of face verification is the generally the same as the one used in fingerprint verification: the camera sensor captures the user’s face, image preprocessing and feature extraction are then performed, and the obtained features are compared against database of known individuals. Deep learning techniques, in particular CNNs, have revolutionized face recognition technology, and led to significant improvements in accuracy. CNNs have generally demonstrated superior performance in processing complex variations in facial appearance, such as pose, illumination, expression, and occlusion. This is especially important since facial features can change due to aging, expressions, or even makeup. A special form of neural network, known as the Siamese network, is particularly useful in face verification. Here, two identical neural networks work in parallel to carry out similarity check between the captured image and enrolled users.
However, basic face recognition systems are highly vulnerable to presentation attacks. In such attacks, an individual is trying to trick the system by presenting fake or manipulated biometric data to gain unauthorized access or perform identity fraud. A typical attack involves using 2D images of the authorized user printed on a paper or displayed on the screen. Some attackers even use masks made of silicone or resin. That’s why many modern face recognition systems employ various countermeasures, such as liveness detection, to ensure they’re not being fooled by a static image or a mask. For example, many modern banking applications now require an additional step during the registration process besides user’s id and current image obtained with the smartphone camera. Examples include blinking detection, head movement checks, or asking users to perform specific facial gestures (smile) or visual dot following on the smartphone screen. Furthermore, techniques for analyzing blood flow patterns or heat signatures on the face, adding additional sensors like depth camera to the system, can help in distinguishing a real person from a replica. For example, Apple’s Face ID (introduced in 2017 with Iphone X) uses TrueDepth camera mounted on the top of the device [3]. TrueDepth camera consists of several sensors including an infrared camera and an infrared projector, enabling the creation of an accurate 3D representation of the user’s face.
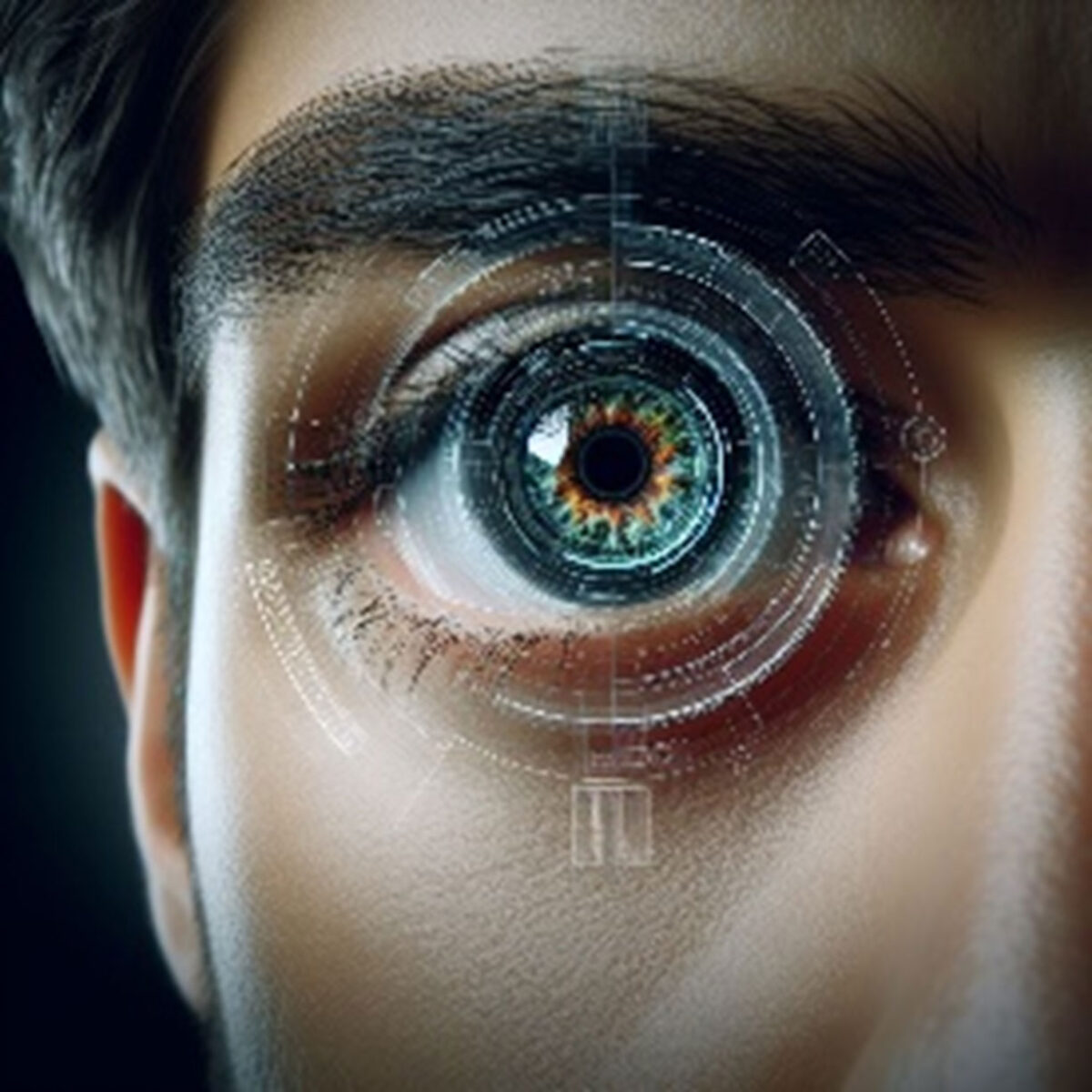
One interesting type of biometric technology is iris recognition. Once a technology only seen in science fiction movies for unlocking doors, iris recognition is gaining wider acceptance in the real-world applications. The texture of the iris is relatively stable across a person’s lifetime leading to very accurate identification compared to other biometrics like fingerprints. However, iris scanner can be more expensive compared to fingerprint scanners. For example, it can be found in high-end devices like Apple Vision Pro mixed-reality headset under the name Optic ID for the purpose of device unlocking or purchases authorization [4]. Usually, near-infrared (NIR) based cameras are used to capture image of an iris due to a better visibility of iris texture in comparison with commercial cameras. The first practical algorithm for iris recognition was proposed in the 1990s by John Daugman. Surprisingly, it is still widely used in iris recognition systems today. Its computational efficiency paired with low false match rate (but keeping false non-match rates at acceptable level) gave it global success and has been adopted in various commercial and government applications. However, modern approaches involving different types of deep neural networks were proposed to improve certain parts of the iris recognition pipeline. Interested reader are pointed to [5] for further details.
What to expect?
The integration of DNNs into modern biometric systems is still evolving, but the potential is huge. Modern DNN are constantly being improving so we can expect even more sophisticated and secure biometric applications. Efficient combination of multiple biometric modalities, such as using fingerprints and iris scans together, can create an even more robust and secure biometric system. Modern DNNs are well-suited to efficiently handle this fusion of data. DNNs can be efficiently trained to detect liveness making biometric systems more resistant to presentation attacks. DNNs can also enable seamless and continuous user authentication based on user’s ongoing behavioural patterns. Nowadays, some of the modern banking applications are tracing your typing style for the purpose of security. Finally, it must be pointed out that your biometric data is highly personal. Regulations and clear guidelines are needed to govern the responsible collection, use, and storage of biometric data.
References
[1] H. Li and R. Ramachandra, “Deep Learning based Fingerprint Presentation Attack Detection: A Comprehensive Survey,” May 2023, Accessed: Jun. 10, 2024. [Online]. Available: https://arxiv.org/abs/2305.17522
[2] Computer Vision Machine Learning Team, “An On-device Deep Neural Network for Face Detection.” Accessed: Jun. 10, 2024. [Online]. Available: https://machinelearning.apple.com/research/face-detection
[3] Apple, “About Face ID advanced technology.” Accessed: Jun. 10, 2024. [Online]. Available: https://support.apple.com/en-us/102381
[4] Apple, “About Optic ID advanced technology.” Accessed: Jun. 10, 2024. [Online]. Available: https://support.apple.com/en-us/118483
[5] K. Nguyen, H. Proença, and F. Alonso-Fernandez, “Deep Learning for Iris Recognition: A Survey,” Oct. 2022, Accessed: Jun. 10, 2024. [Online]. Available: https://arxiv.org/abs/2210.05866
Author: Associate Professor Ratko Grbić, PhD
Text and/or images are partially generated by artificial intelligence